Weak-to-strong generalization
A new research direction for superalignment: can we leverage the generalization properties of deep learning to control strong models with weak supervisors?
I'm very excited and proud to share some of the work we've been up to: a new research direction, with some promising initial results, for aligning superhuman AI systems.
OpenAI blog post - Paper (oral at ICML)
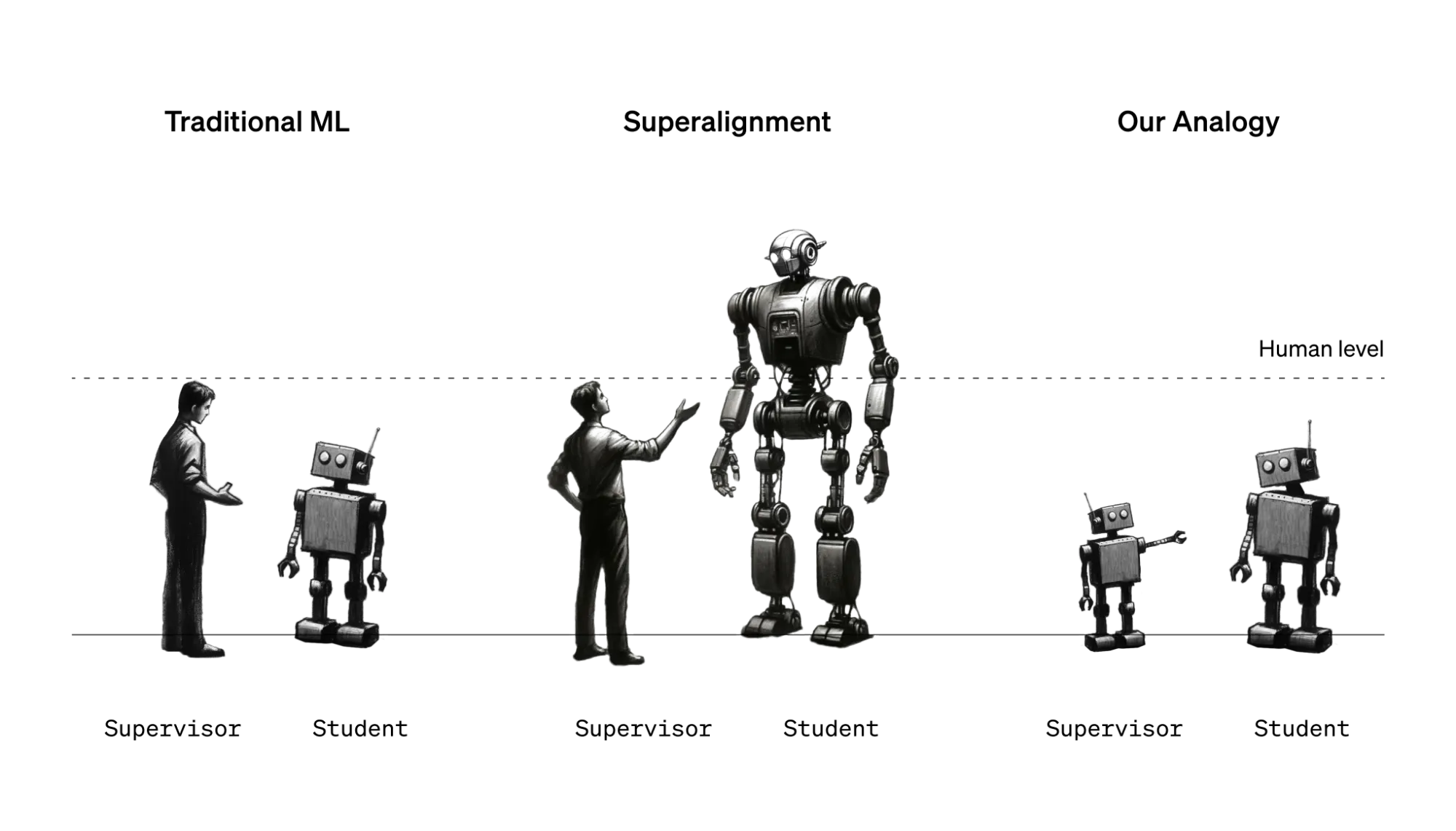
A core challenge for aligning future superhuman AI systems (superalignment) is that humans will need to supervise AI systems much smarter than them. We study a simple analogy: can small models supervise large models? We show that we can use a GPT-2-level model to elicit most of GPT-4’s capabilities—close to GPT-3.5-level performance—generalizing correctly even to hard problems where the small model failed. This opens up a new research direction that allows us to directly tackle a central challenge of aligning future superhuman models while making iterative empirical progress today.
Intuitively, superhuman AI systems should "know" if they're acting safely.
— Leopold Aschenbrenner (@leopoldasch) December 14, 2023
But can we "summon" such concepts from strong models with only weak supervision?
Incredibly excited to finally share what we've been working on: weak-to-strong generalization. 1/https://t.co/FiFGhrqqE0 pic.twitter.com/XyMO1Kjj5o
FOR OUR POSTERITY Newsletter
Join the newsletter to receive the latest updates in your inbox.